Human genetics and drug development
Growing evidence that human genetics can double or even triple drug development success rates
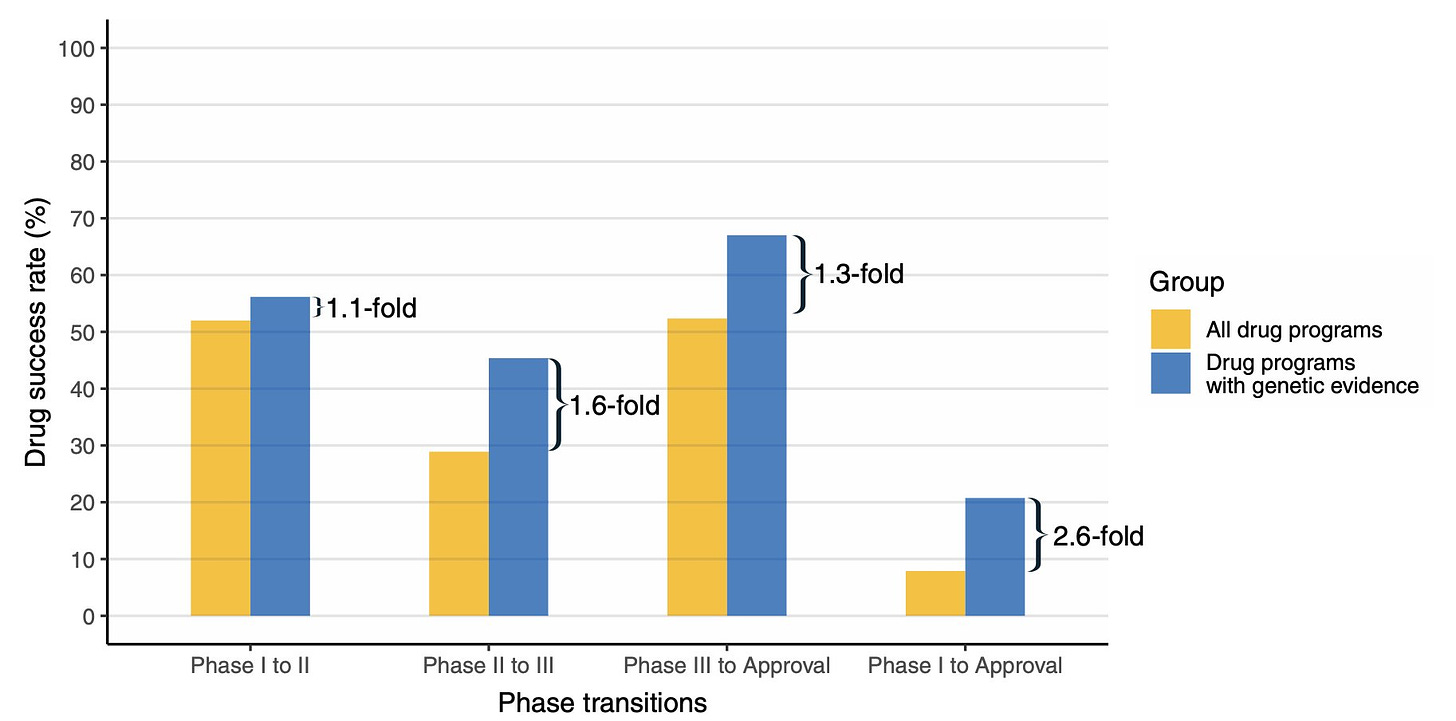
Drug development is a risky business. Less than 1 out of 10 drugs that enter clinical development succeeds (success defined as getting an FDA approval and entering the market). Because of this extremely low success rate, the cost of developing a drug has skyrocketed. The expectation is that to land on one successful drug, you should work on at least 10, to increase your odds of succeeding. And landing on that one success will offset all the money wasted in developing the failed drugs. This current business model demands huge investment to run a drug business, often in the range of billions of dollars. In a such a scenario, strategies that can push the base success rate beyond 10% will prove highly valuable for drug companies. One such strategy is to embrace human genetics.
Recently I contributed a 'Views and News' article to GEN Biotechnology journal on invitation from the journal's executive editor Kevin Davies. The commentary was on an article published recently in Nature by Matt Nelson and colleagues on the value of human genetics to improve drug success.

Nelson is well known in the field for his landmark article in Nature Genetics from 2015. It was the first empirical demonstration that human genetic evidence can increase the odds of drug success. Through a systematic analysis of public and proprietary databases, Nelson et al. arrived at the conclusion that prioritizing drug targets based on human genetics evidence can nearly double the success rate. The paper was a hit. The impact it had on both industry and academia was beyond Nelson's or anyone's expectation. It became an essential reference in investment pitches made to biotech VCs. It has probably influenced many big investment decisions in the past decade.
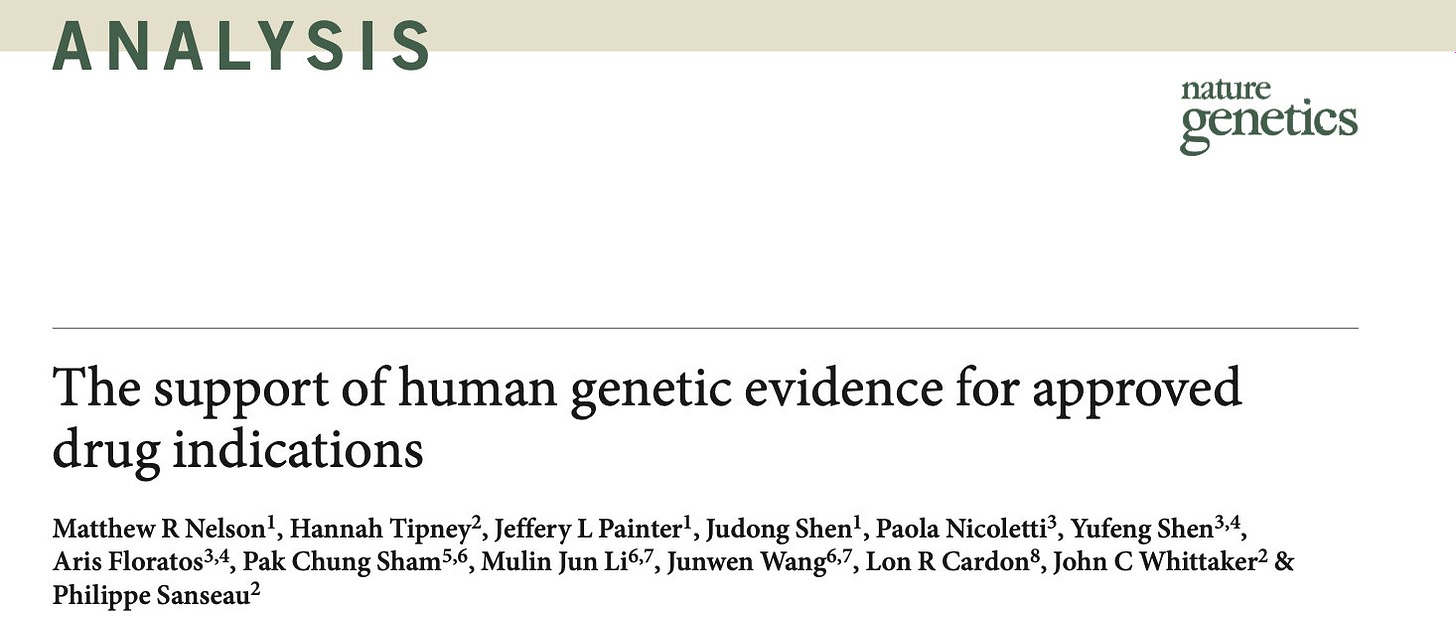
Nelson and colleagues' recent work is a follow-up of their 2015 analysis. The core idea of the analysis in both their articles is to curate a list of drug programs that are in various stages of development and evaluate how often programs backed by human genetics succeeded compared to others. It's important to understand what, according to the authors, qualifies as a "human genetics evidence". If the gene corresponding to the drug (that is, the drug works by targeting either the gene or its product) has any evidence of association with the indication in the literature, it is considered to have a human genetics evidence. If there is no published human genetic literature linking the drug target to its indication, it doesn't mean human genetics favors against the drug. It only means there is no published evidence at the moment, but it might surface later as new studies get published. And that is the motivation behind Nelson and colleagues recently following up on their past analysis using latest data. This work was led by Eric Vallabh Minikel from Broad Institute in collaboration with Nelson and two other industry scientists.
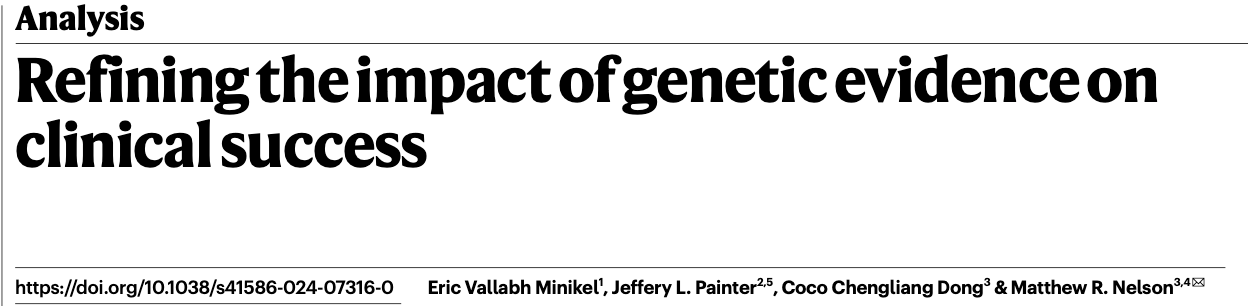
Using a proprietary database of drug programs curated since 2000 by Citeline Pharmaprojects, Minikel et al. made a list of ~30,000 target-indication pairs. Using publicly available human genetics databases, they then made a list of ~82,000 gene-trait pairs. Overlapping these two, they found a little more than 2000 target-indication pairs that also had matching gene-trait pairs, which is a mere 7.3% of the total target-indication pairs. This is their main group, that is, the group of drugs with a supporting human genetic evidence. And the rest of 92.7% of target-indication pairs are their reference group, that is, target-indication pairs with no human genetic evidence yet. Here, absence of evidence doesn't mean evidence of absence. Many of the target-indication pairs in reference group can switch to main group as new studies get published, or new databases get released.
So, what did the authors find? The relative success rate of drug programs in the main group (with human genetic evidence) to move from phase 1 all the way to FDA approval was 2.6-fold higher than the drug programs in the reference group (without human genetic evidence). This effect size is 30% more than their 2015 estimate. This is interesting, why? Because it says that the human genetic discoveries are not saturated yet. As more findings get published, the value of human genetics in predicting drug success will further increase. Perhaps that realization is what motivated Nelson to start his company, Genscience. According to the website, the goal of the company is to "integrate world-class genetic insights into drug discovery and development decisions with differentiated expertise, platforms, and analytics." If you'd like to learn more about Nelson and his new company, check episode 118 of The Genetics Podcast, hosted by Patrick Short (YouTube, Apple podcast).
Clearly, Nelson has pioneered a research path that is inspiring many. Recently, 23andMe published a preprint on a similar analysis. Given the recent unfavorable market situation, the company has made a brilliant move to showcase the value of their in-house genetic database. Following the footsteps of Nelson, 23andMe scientists (Wang et al.) combined the genetic association data based on 15 million 23andMe participants (who volunteered to participate in research) with Citeline Pharmaprojects database and evaluated the success rate of drug programs with human genetics support. They arrive at a similar conclusion as their predecessor—drug programs with human genetic support based on self-reported phenotypes are 2 to 3 times more successful than drug programs without human genetic support. Here, the phrase 'self-reported' is notable. Because one criticism that 23andMe has often received in the early years is the questionable value of self-reported phenotypes. And that is one of the reasons 23andMe has been extensively collaborating with academic researchers to show that self-reported phenotypes can produce reliable genetic results as clinical phenotypes when collected at scale. And proving this was an important requirement for GSK to pay 300 million dollars to 23andMe for an exclusive four-year access to 23andMe genetic database in 2018. 23andMe’s recent preprint and its eventual publication will become a key document in all their future deals with drug companies who would want to incorporate 23andMe genetic data into their drug pipelines.
One of the interesting findings in the preprint is the difference in the impact of rare vs common variants on drug success. Minikel et al. argue that minor allele frequency or effect size did not influence the success rate, and that common variants predicted drug success just as efficiently as rare variants, and small effect size variants predicted drug success just as efficiently as large effect size variants. But Wang et al. show otherwise. When stratified by minor allele frequency, genetic evidence based on rare variants seem to double the impact compared to genetic evidence based on common variants, the same true for effect size. Note, these insights are based on imputed rare variants. 23andMe doesn't sequence their participants, they only genotype and impute the variants based on a reference panel. But when you're imputing variants in millions of participants, even rare variants with modest imputation accuracy can yield accurate results, as the sheer size of the data compensates for the reduced imputation accuracy. For example, if you're predicting a rare variant to be present in 20 individuals, of which only 15 are true carriers, you won't have the power to identify genetic associations. But when you are predicting a rare variant to be present in 200 individuals, of which 150 are true carriers, you now start to pick up genetic associations. With further increase in sample size, you gain more power.
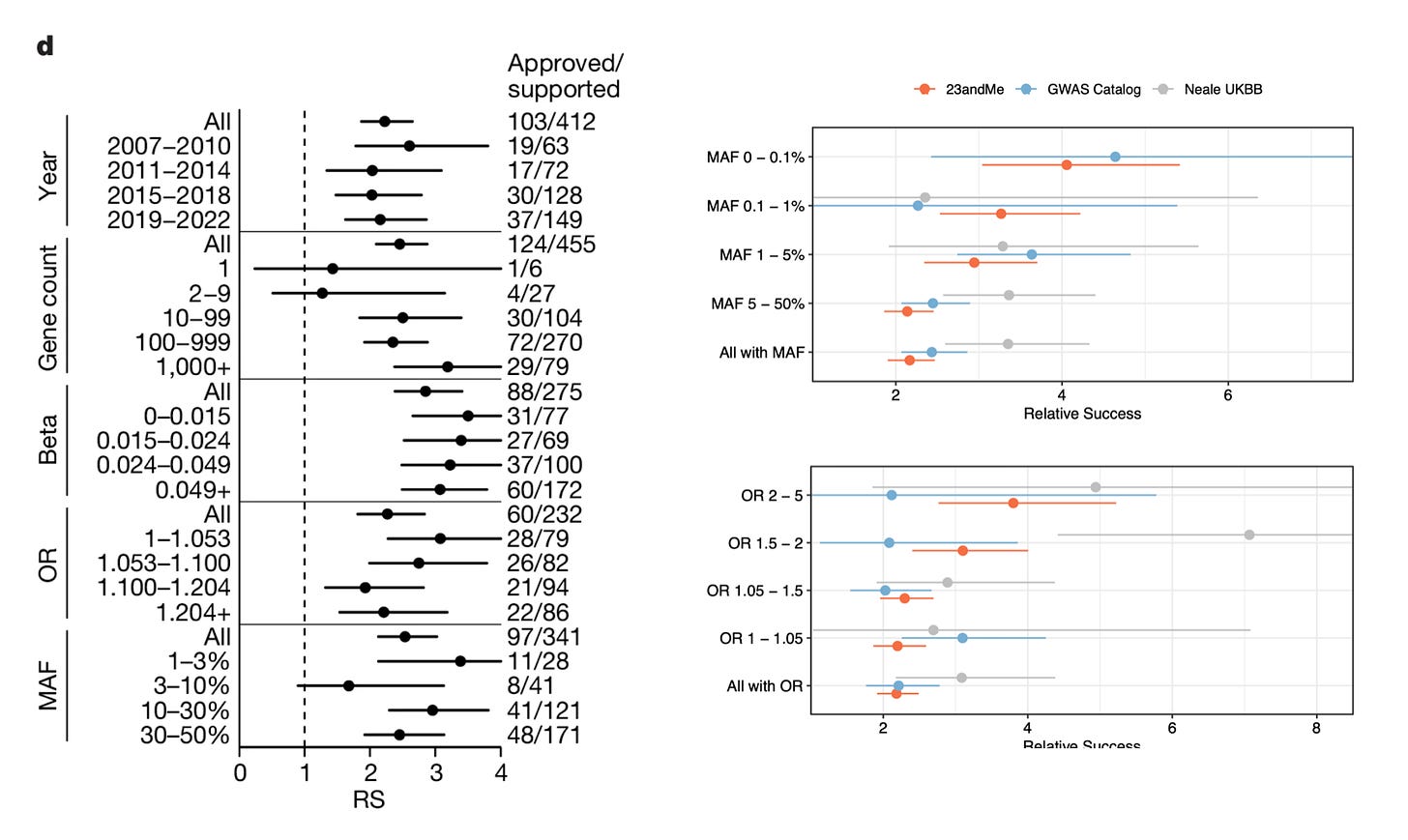
Although one might immediately assume that rare variants perform better than common variants because rare variants have larger effect size, and genes with larger effect size are better drug targets than those with smaller effect size. Remember, here we are not using human genetics to discover targets. We are simply using it to increase our confidence on already discovered targets. For such purposes, effect size shouldn’t matter. Effect sizes are reflection of the severity of the genetic variant. A loss of function variant will of course have a larger effect size than a missense variant or an eQTL variant. But as long as the genetic association establishes the link between target and indication, the effect size shouldn’t matter. At least that is the current understanding.
So, then why rare variants perform better in Wang et al’s analysis? It’s because rare variants precisely pinpoint causal genes at the locus of association, but common variants don’t. Both Minikel et al. and Wang et al. are on agreement on this. Minikel et al. show this by using a metric created by Open Targets called locus-to-gene (L2G) score. L2G score derives information from multiple sources such as distance from the index variant, coding variants in linkage disequilibrium, effect on gene expression (eQTLs), overlap with epigenetic marks etc. and predicts the likelihood of a gene to be causal. The authors show that as the L2G score increases (meaning, as the confidence in the causality of the genes increases), the prediction performance increases. Similarly, Wang et al. demonstrate the same, based on their own V2G score estimates. Further, they also show that success rates are higher when causal genes were assigned based on allelic series (meaning, identifying independent variants in the same locus, all linking to the same gene), rare variant burden tests and coding variants in comparison to other methods such as eQTLs, nearest gene etc. We know that former list are better predictors of causal genes than the later.
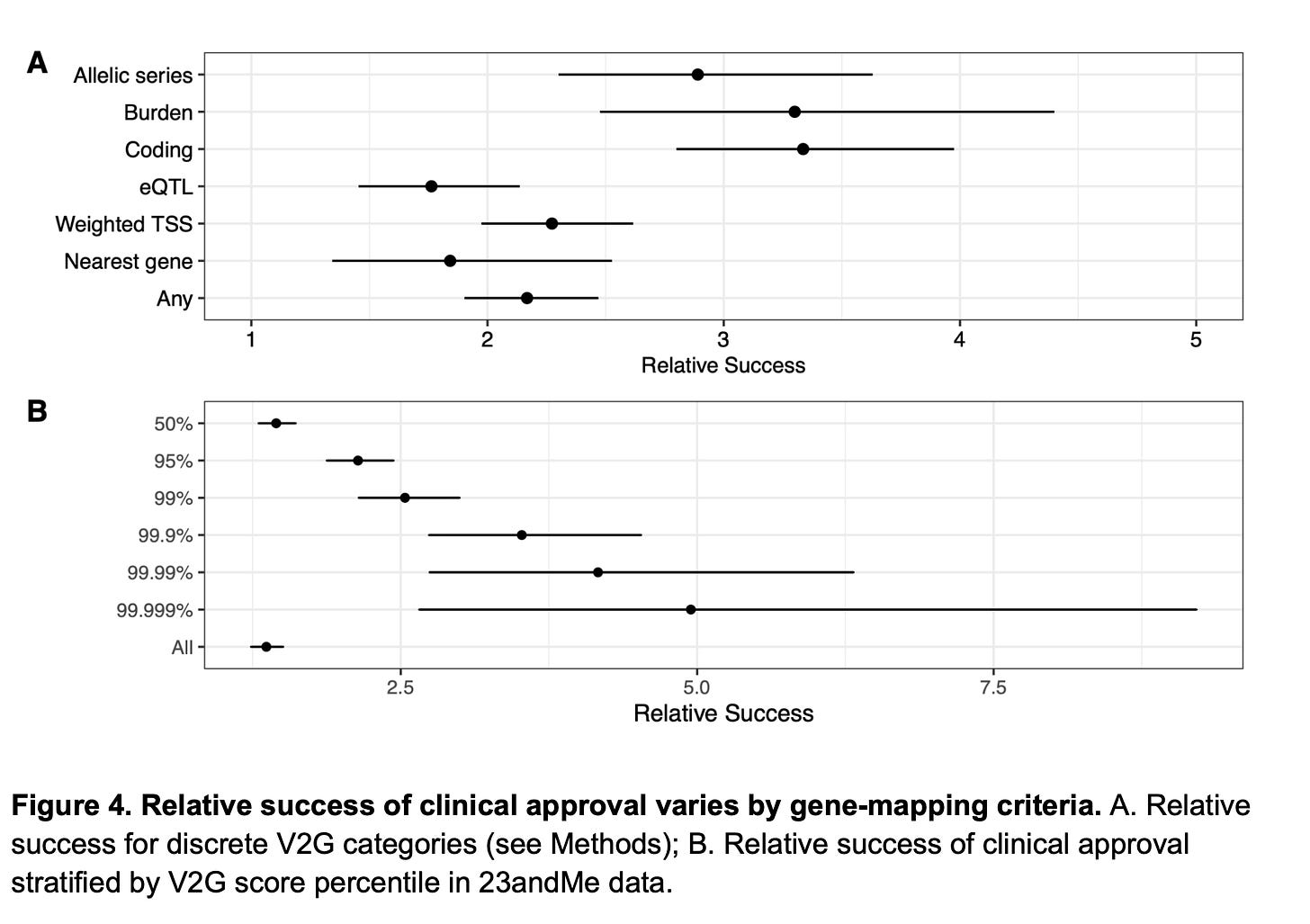
So, it is becoming clear that we will be seeing more analyses predicting drug program success with human genetics data periodically in the future, either from Genscience or other companies like 23andMe with their own in-house genetic data. But at the end of the day, we shouldn't forget that all these analyses are retrospective in nature where we draw conclusions on past successes. Importantly, it is a biased analysis as we are looking at only reasons for successes but not reasons for failures. Many in the field argue that the real value of human genetics is not to foretell which drugs will succeed but to predict which ones won’t. However, it’s difficult to test this as it’s difficult to confidently tell that a gene A is not associated with a trait X (there could be many reasons why a variant is not associated with a trait). But it’s easy to tell that the gene A is associated with the trait X. So, we can only empirically reason on past successes but not failures.
An ideal analysis should be a randomized trial where you randomly select two groups of drug candidates. In the first group, prioritize drug candidates for clinical development with insight from human genetics, and in the second group, do the same but without any insight from human genetics. Which group will succeed more? Such an experiment is unrealistic. But human genetics is now becoming an important layer of evidence to prioritize drug programs in many companies. Based on the experiences of such companies, we will know the real world impact of human genetics in improving the drug success rate. When I tweeted about my article recently, John Maraganore, the former CEO of Alnylam, said they achieved 60% success rate (that is 6 times the base rate) when using genetics to prioritize their RNAi drugs. Amazing isn’t it? There is no better time than now to be working and investing in human genetics.
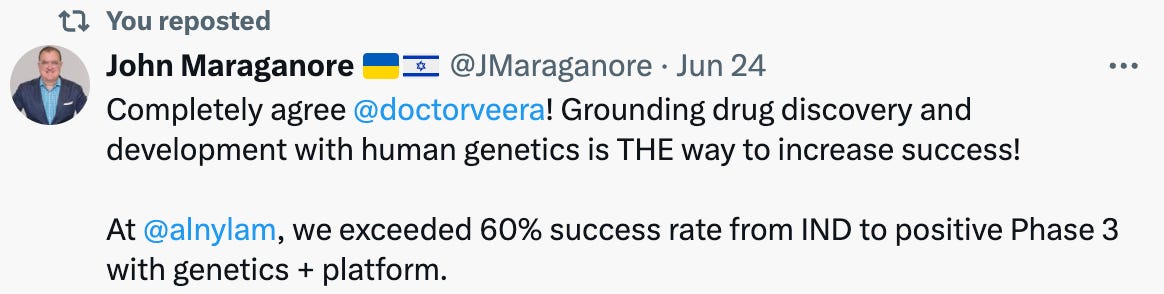