Genomes vs Exomes
Whole genome sequencing vs whole exome sequencing: which one is cost-effective for gene discovery?
Regular readers of my Substack will know that I am a noncoding genome enthusiast. I get excited every time I read about a new noncoding discovery, and I highlight such findings in the Substack post. However, as an industry scientist working on drug discovery, if I am asked to choose between whole exome sequencing (WES) and whole genome sequencing (WGS), my money would be on WES. My colleagues at Regeneron Genetics Center (RGC) feel the same.
RGC was one of the eight pharmaceutical companies that invested in the whole exome sequencing of 500,000 UK Biobank research participants. But when industry giants later joined hands to throw money on whole genome sequencing the full UK Biobank, RGC opted out. The leadership, which includes pioneers in human genetics, strongly felt that WES provides more bang for the buck than WGS. They even assigned a team to empirically make this case. The results of their study are now published in Nature Genetics. In the study, Gaynor et al. ask how many more genetic associations can be found by WGS compared to WES and arrive at a sobering answer: not that many.
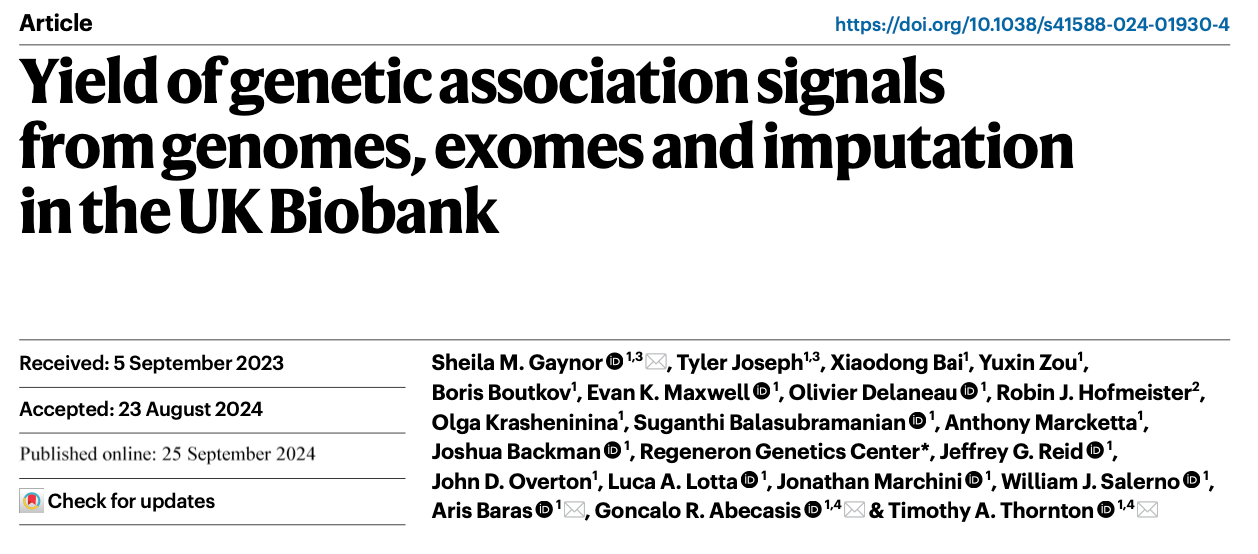
The current standard in the field to perform genome-wide association study (GWAS) is by combining array genotyping with WES. The array genotyping measures a sparse set of variants (~500,000) distributed across the genome, which are then used to impute unmeasured variants (~10M to 15M) using linkage disequilibrium (LD) maps. This approach helps to confidently identify only common variants (minor allele frequency >1%) as imputation accuracy drops at lower allele frequencies due to poor LD. So, researchers go for sequencing technology to measure rare variants. WES helps find rare variants from the coding regions, which span around 1-2% of the genome. So, the combination of common variants across the genome and rare variants across the exome has been the work horse of the field so far. So, the extra information that WGS provides is rare variants from the noncoding genome. Since the noncoding genome comprise more than 98% of the genome, there has been understandably high expectations for potential genetic discoveries from noncoding rare variants. In their analysis, Gaynor et al. specifically evaluated the yield of new genetic signals from noncoding rare variants by making a head-to-head comparison between WES+IMP and WGS.
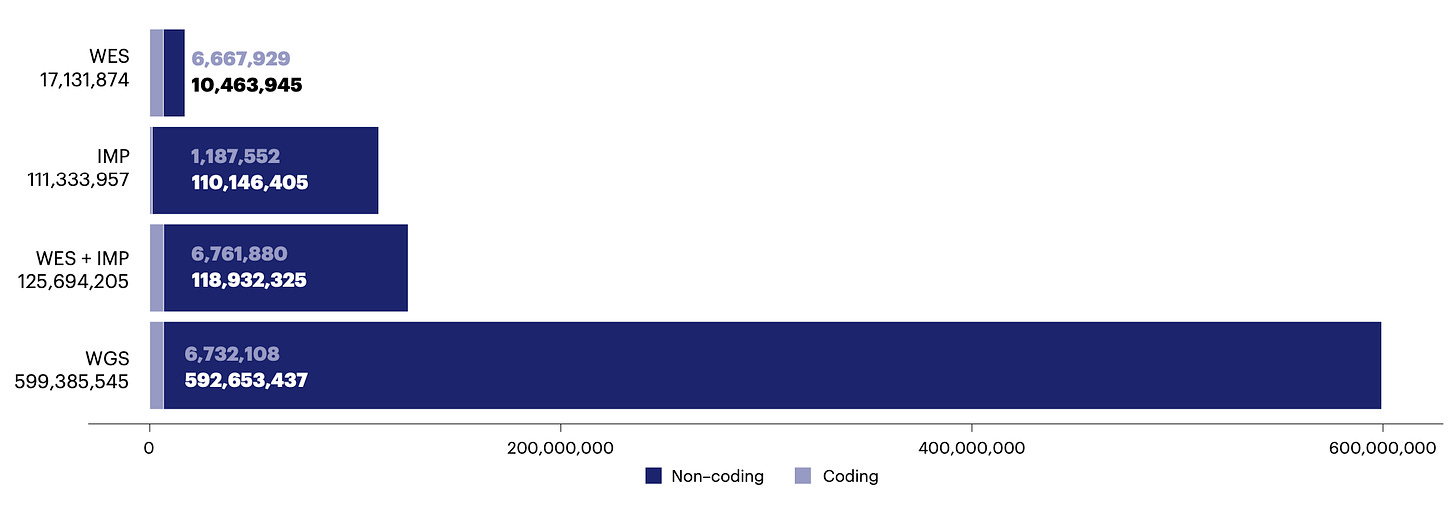
The authors analyzed the genetic data of 150k UK Biobank research participants. As expected, they over all found more variants using WGS than WES+IMP. While WES+IMP identified 125M variants, WGS identified 600M variants. But the important question is if this 5x more variant yield will translate to 5x more discoveries. When the authors performed GWAS of 100 phenotypes, they found that the yield of genetic associations were similar between the two approaches. This is not surprising because most of the extra variants that WGS capture are rare variants, particularly singletons (i.e., private variants observed in only one individual). Nearly half of the 600M variants identified by WGS are singletons, whereas only 7% of the 125M variants identified by WES are singletons.
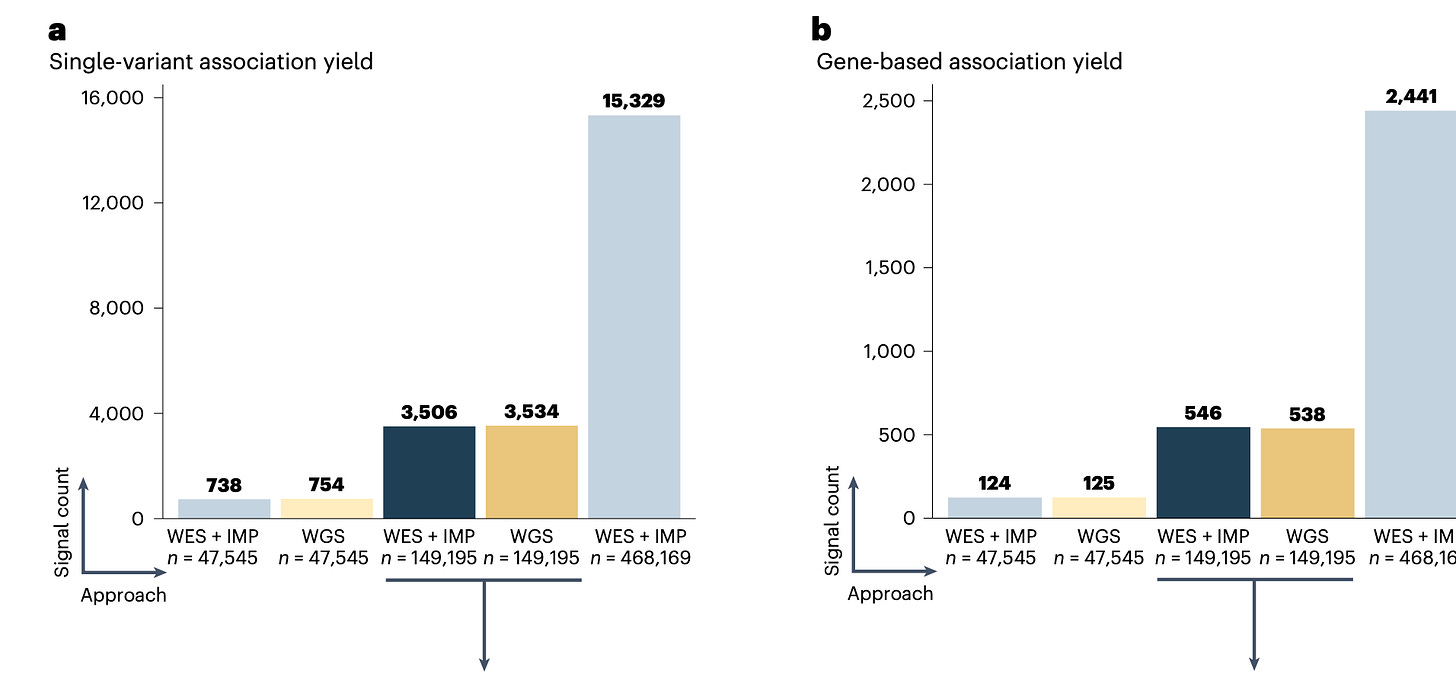
To identify genetic associations with rare variants, we need sufficient number of carriers. So, at the single variant level, the singletons and most of the ultra rare variants will not yield any significant genetic associations. In the WES analysis, we circumvent this low carrier count problem through a burden approach. We collapse rare variants across each of the genes into a single group. For example, if there are 20 rare predicted loss of function (pLOF) variants in, let's say, gene A and each of the variants are seen only in one or a few individuals, we pool all the carriers together resulting in 50 to 100 carriers, thereby boosting statistical power to test genetic associations. However, this collapsing technique doesn't work for noncoding variants, at least, not as as straightforward as it is for coding variants. While dealing with coding variants, we have well defined discrete genome boundaries defined by gene sequence and well defined variant classes such as loss of function, missense variants etc. In the case of noncoding variants, we do not have well defined boundaries and variant effect classes. The existing noncoding annotations like promoters, enhancers, 3' and 5' untranslated regions (UTRs) etc. are blurry and highly context specific. These limitations substantially reduce the number of meaningful discoveries that one can make sequencing the noncoding genome. A good example is a recent paper on noncoding rare variant associations with height, published by researchers at the University of Exeter in the UK.
Hawkes et al. analyzed WGS data of more than 300k individuals from UK Biobank, TOPMed and All of Us cohorts and tested the associations of rare coding variants with height. They used both single variant and collapsing approaches for association testing. After excluding known associations, the authors ended up with mere 29 associations. Only a few of these 29 associations implicate any genes. Some of the associations are of course interesting. They include noncoding variants near genes well associated with height, for example, GHRH (growth hormone releasing hormone), and noncoding variants implicating novel genes in the biology of height, for example, HMGA1 (high mobility group A1). But the question is, are these handful of discoveries worth spending money to sequence the whole genome of 330k participants, when you could use the same money to sequence perhaps 3 to 4 times more participants?
In their study, Gaynor et al. demonstrate the disproportionately higher yield of genetic signals with increasing sample size. While the authors did many interesting comparisons, I'll focus here on one finding that signifies the importance of sample size in gene discovery. The authors compared two extreme sample sizes of ~47k and ~470k. When testing genetic associations using these sample sizes, the authors found that a 10-fold increase in sample size yielded approximately 20-fold increase in genetic associations. Coming back to height, my colleagues have been working on an exome-wide association study (ExWAS) of height in > 1 million individuals. The preliminary findings were presented at last year’s American Society of Human Genetics (ASHG) meeting, and updated results are planned to be presented at this year’s ASHG. I recommend checking the presentation to appreciate the power of sample size in rare variant discovery.
I may sound bearish on WGS. But I am not. I believe WGS has an enormous potential in advancing genetic discoveries. But it will take time. As much as I am against the idea of spending money on WGS for drug discovery, I couldn't be more excited about the fact that there are people who have invested generously in whole genome sequencing the entire UK Biobank. The below tweet from Daniel McArthur, a prominent leader in human genetics, summarize how I and many of my colleagues felt.
One thing that I'll closely watch is what new findings emerge from UK Biobank WGS data. There is no doubt that many fascinating discoveries will be made, as illustrated by deCODE Genetics in their flagship paper on the initial analysis of the first 150,000 genomes. I've highlighted in my past posts some of the fascinating discoveries reported in the paper. As people play around with the full UKB WGS dataset, more such findings will trickle down over the next years.
Personally, I am more interested in the potential of noncoding variants in advancing drug development as I've explained in a recent post on the discovery of a noncoding enhancer variant linked to a Mendelian form of heart disease. Particularly, I am curious about the value of noncoding variants to inform consequences of disrupting genes that are constrained for coding variants. Genes that play critical roles during early development are often intolerant to damaging mutations. Hence, we see rarely any carriers of pLOFs or deleterious missense variants in such genes in the general population. I expect noncoding variants that disrupt such genes, perhaps only in specific tissues or during the late stages of development, might surface in the future GWAS based on WGS data. For example, in the height paper by Hawkes et al., the authors write that the new gene, HMGA1, that they have linked to height via noncoding variants has no coding variant association with height. They discuss that this may be because the gene is mutationally constrained. The authors found that among the 330k UK Biobank participants they analyzed, only one carried a pLOF in HMGA1. So, it may be the case that HMGA1's crucial role in early development may have previously obscured its connection to height, which is now being revealed through the study of noncoding variants.
Speaking of WGS, I should mention about its value in the rare disease space. I've repeatedly mentioned this in the past: important noncoding discoveries will often emerge not from analyses of hundreds of thousands of individuals from large biobanks, but from handful of individuals with rare diseases. We've seen many examples so far (HK1, CHD2, KCNB1, ASIP and RNU4-2). Although these examples have exemplified the potential of WGS in rare disease diagnosis, it is challenging to confidently say that WGS is more cost effective than WES for diagnosing rare diseases. Few recent studies have shown mild to moderate higher diagnostic yield for WGS compared to WES. However, given the multiple fold cost difference between WES and WGS, one would argues that one can provide genetic diagnoses to more families by simply opting to choose WES. Perhaps, WGS could be reserved only for families unsolved using WES. But then there are example such as RNU4-2 that clearly make the case that many common causes of neurodevelopmental diseases (NDDs) remain undiscovered because of the long standing bias towards coding genome. Mutations in RNU4-2, encoding a small nuclear RNA involved in gene splicing, were found to explain nearly 0.4% of all NDDs. That is a big number and makes a strong case for WGS for rare disease diagnosis.
As you can see, unlike common diseases, the question of whether WES is more cost effective than WGS is more debatable when it comes to rare diseases. There are valid arguments on both sides. Nevertheless, the field is quickly moving towards WGS in the rare disease space. Furthermore, the cost of WGS is also continuing to drop. So, WGS will become the default choice of sequencing some day. But it’s difficult to predict how far that day is from now.